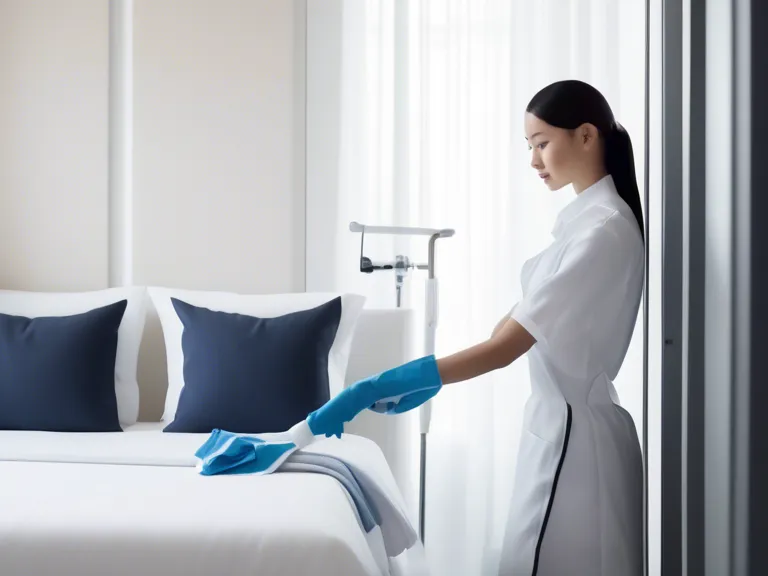
Introduction
Housekeeping tasks can often be time-consuming and tedious, taking away valuable time that could be spent on more meaningful activities. However, with the advancements in technology, specifically machine learning, it is now possible to streamline and optimize various housekeeping tasks to make them more efficient and effective.
Understanding Machine Learning in Housekeeping
Machine learning is a branch of artificial intelligence that enables systems to learn and improve from experience without being explicitly programmed. In the context of housekeeping, machine learning algorithms can be utilized to analyze data, identify patterns, and make predictions to automate and optimize various tasks.
Benefits of Using Machine Learning in Housekeeping
Time Efficiency: Machine learning algorithms can analyze patterns in housekeeping tasks and suggest the most efficient ways to complete them, saving time for the housekeeper.
Resource Optimization: By analyzing data on resource usage, machine learning can help in optimizing the use of cleaning supplies, energy, and water, leading to cost savings.
Personalized Recommendations: Machine learning can analyze preferences and habits to provide personalized recommendations on cleaning schedules, products, and techniques tailored to individual households.
Predictive Maintenance: Machine learning algorithms can predict when household appliances or equipment may require maintenance or replacement, helping in preventing breakdowns and prolonging their lifespan.
Applications of Machine Learning in Housekeeping
Smart Cleaning Devices: Machine learning can be integrated into smart cleaning devices such as robot vacuums to optimize cleaning paths based on room layouts and usage patterns.
Energy Efficiency: Machine learning algorithms can analyze energy consumption patterns to suggest ways to reduce energy usage in the household, such as optimizing heating and cooling systems.
Waste Management: Machine learning can identify patterns in waste generation and disposal to suggest recycling strategies and reduce overall waste production.
Challenges and Considerations
Data Privacy: Collecting and analyzing household data for machine learning applications raises concerns about data privacy and security. It is essential to ensure that data is handled responsibly and ethically.
Adaptability: Machine learning algorithms may require frequent updates and fine-tuning to adapt to changing household dynamics and preferences.
Integration: Integrating machine learning solutions into existing housekeeping routines and technologies may require training and support to ensure successful implementation.
Conclusion
In conclusion, machine learning offers significant potential to streamline housekeeping tasks and improve efficiency in households. By harnessing the power of data analysis and predictive algorithms, housekeepers can optimize their cleaning routines, save time and resources, and enhance the overall quality of living spaces. Embracing machine learning in housekeeping can lead to a more sustainable and enjoyable home environment for residents.
drinkganbei.com
mendenhallnews.com
nathaliemoliavko-visotzky.com
nationalinfertilityday.com
wide-aware.com
ashleymodernfurniture.com
babylonbusinessfinance.com
charliedewhirst.com
christianandmilitaryhats.com
hypnosisoneonone.com
icelandcomedyfilmfestival.com
kayelam.com
mlroadhouse.com
mumpreneursonline.com
posciesa.com
pursweets-and.com
rgparchive.com
therenegadehealthshow.com
travelingbitz.com
yutakaokada.com
22fps.com
aarondgraham.com
essentialaustin.com
femdotdot.com
harborcheese.com
innovar-env.com
mercicongo.com
oabphoto.com
pmptestprep.com
rmreflectivevest-jp.com
tempistico.com
filmintelligence.org
artisticbrit.com
avataracademyagency.com
blackteaworld.com
healthprosinrecovery.com
iancswanson.com
multiversecorpscomics.com
warrenindiana.com
growthremote.com
horizonbarcelona.com
iosdevcampcolorado.com
knoticalpr.com
kotaden.com
la-scuderia.com
nidoderatones.com
noexcuses5k.com
nolongerhome.com
oxfordcounselingcenter.com
phytacol.com
pizzaropizza.com
spotlightbd.com
tenbags.com
thetravellingwilbennetts.com